Random Research highlight: The Consensus Connectome Dynamics and Directed Structural Connectomes: The human braingraph is the object of an intensive research today. The advantage of the graph-approach to brain science is that the rich structures, algorithms and definitions of the 275 years old graph theory can be applied to the anatomical networks of the connections of the human brain. In these graphs, the vertices correspond to the small (1-1.5 square cm) areas of the gray matter, and two vertices are connected by an edge, if a diffusion-weighted imaging workflow finds fibers of axons, running between those small gray matter areas. In a previous work we have reported the construction of the Budapest Reference Connectome Server from the data of the Human Connectome Project. The server generates consensus braingraphs: braingraphs of connections that are present in at least k graphs out of the n=418, for any value of k = 1, 2, ..., 418. When the value of k is changed from k = 418 through 1 by moving a slider at the webserver from right to left, certainly more and more edges appear in the consensus graph. The astonishing observation is that the appearance of the new edges is not random: it is similar to a growing shrub or tree. We refer to this phenomenon as the Consensus Connectome Dynamics. We hypothesize that this movement of the slider in the webserver copies the development of the connections in the human brain in the following sense: the connections that are present in all subjects are the oldest ones, and those that are present only in a decreasing fraction of the subjects are gradually the newer connections in the individual brain development. An animation on the phenomenon is available here. Based on this observation and the related hypothesis, we can assign directions to most of the edges of the structural connectomes, first in the literature.
PLoS One, 11(6): e0158680 doi:10.1371/journal.pone.0158680.
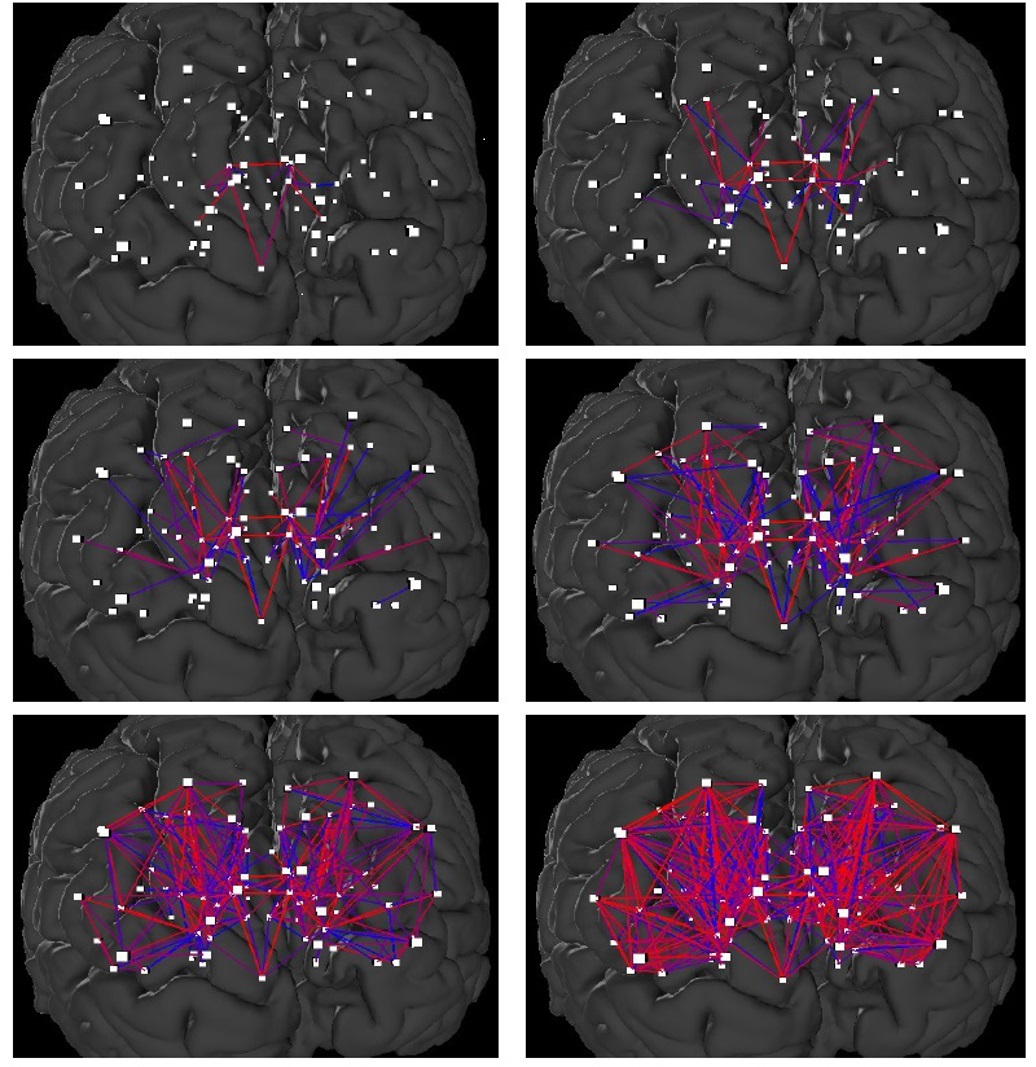